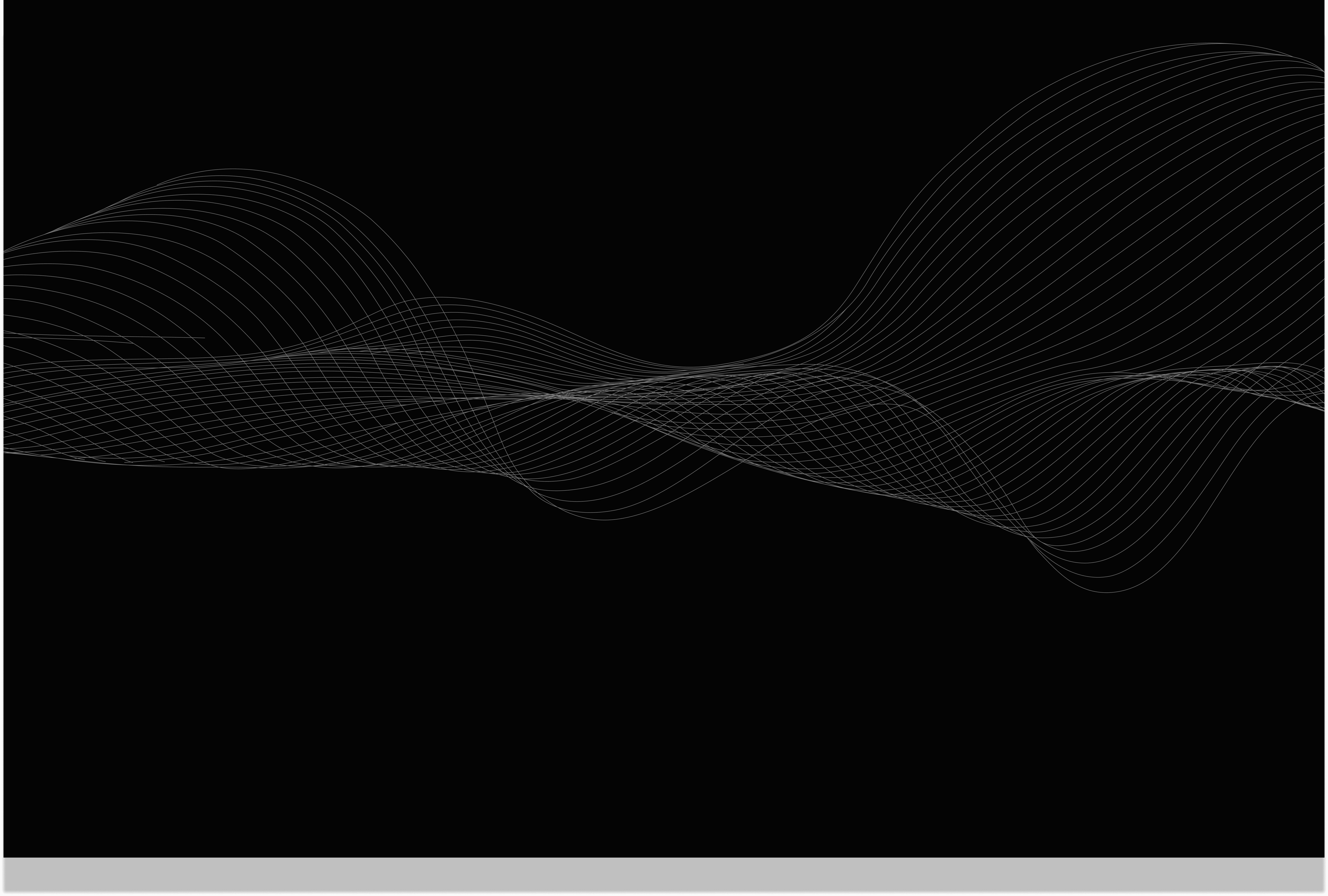
Voice AI Platforms in 2025: A Conversation with Vapi Founder Jordan Dearsley
Mar 27, 2025
In the rapidly evolving landscape of voice AI technology, understanding the current state of platforms and their future trajectory is essential for businesses looking to leverage these powerful tools. We recently had the opportunity to speak with Jordan Dearsley, founder of Vapi, a leading voice AI platform, about where voice AI stands today and where it's headed. Vapi has quickly established itself as one of the most developer-friendly voice AI platforms on the market, making it an ideal case study for understanding the broader voice AI ecosystem.
The Evolution of Voice AI Platforms
The voice AI ecosystem has undergone tremendous growth since its early days in late 2023. As Jordan explains, when Vapi first emerged around August-September 2023, they were among just a handful of startups "tinkering with voice-related stuff." These pioneering companies were what Jordan refers to as "the innovators" on the adoption curve.
Initially, Vapi's primary focus was on solving technical challenges, particularly latency. Getting response times low enough for natural conversation was a significant hurdle and became a key differentiator for Vapi in the early market. Jordan notes that Vapi's emphasis on reducing latency was critical: "that latency piece was really our differentiator at the time and the reason that people would use us rather than roll it themselves." Most of Vapi's initial customers were small, technical teams with high tolerance for occasional downtime, so reliability at scale wasn't yet a major concern.
The Inflection Point
The real turning point for voice AI came in early 2024, particularly around March, when venture funding began flooding into voice AI startups. This capital influx enabled these companies to evolve from pre-seed experiments to funded seed-stage companies delivering real business value through phone interactions.
Jordan notes that OpenAI's GPT-4 announcement created broader awareness about voice AI's potential, prompting both startups and established companies to explore voice technologies. By October 2024, enterprises began seriously considering voice AI integration, seeking solutions that could work reliably at scale.
Platform Selection: Build vs. Buy Considerations
For companies considering voice AI implementation, the build-versus-buy decision has evolved significantly. While building in-house made sense for some early adopters, Jordan observes that today, "it just doesn't make sense for the time investment" given the availability of robust, out-of-the-box solutions like Vapi.
Jordan strongly emphasizes that "infrastructure itself is not something that people should spend time on" - particularly for verticalized voice AI startups with limited resources. He advises against building voice infrastructure from scratch unless voice technology is a core competitive advantage for your business: "I don't think anyone that's not running real-time audio systems should be running real-time audio systems."
When making the build vs. buy decision, Jordan recommends taking a careful look at documentation, evaluating feature-by-feature tradeoffs, and planning for future needs: "make sure that is going to be supported." For the rare case where specialized functionality is needed (like custom turn-taking behavior with background noise), building in-house might make sense, but Jordan notes these scenarios are "quite rare."
Jordan estimates that only about 10% of companies genuinely benefit from building their own voice stack, with most others better served by focusing on their specific vertical and customer needs while leveraging existing platforms. This insight has shaped Vapi's approach to providing a developer-friendly platform that handles the complex infrastructure requirements so companies can focus on their core business value.
Platform Differentiation in 2025
When evaluating voice platforms, companies should consider:
Technical needs: Some platforms cater to technical developers who need granular control, while others offer no-code/low-code solutions for operations teams without engineering resources.
Configuration requirements: Different platforms offer varying levels of customization for voice agents.
Scalability: Enterprise-grade scaling capabilities matter for businesses anticipating high call volumes.
Future planning: Consider what models and features the platform will support as technology evolves.
Jordan positions Vapi as a developer-focused solution offering substantial control without requiring the complexity of fully custom infrastructure. "We're definitely more for a technical developer," Jordan explains when describing Vapi's target audience. This approach makes Vapi particularly well-suited for technical teams building production voice applications who need powerful capabilities but don't want to invest in building and maintaining voice infrastructure from scratch.
The Voice-to-Voice Model Revolution
Voice-to-voice models represent the next frontier in voice AI technology. Vapi has recently integrated support for Sesame, with Jordan proudly announcing during the podcast: "we launched Sesame on the [Vapi] platform today." While noting it's still in beta, this addition to Vapi's capabilities demonstrates the platform's commitment to staying at the cutting edge of voice technology.
These voice-to-voice models promise more natural interactions and easier multilingual support, with Jordan predicting they could drive "maybe 20% adoption" on the Vapi platform by the end of this year. The multilingual capabilities and natural sound quality make these models particularly promising for certain use cases.
However, challenges remain in configuration, integration with existing telephony systems, and scaling infrastructure to handle millions of calls. Jordan emphasizes that even with speech-to-speech models, the need for robust infrastructure and expertise in scaling real-time audio systems will remain critical—a need that Vapi has specifically engineered its platform to address.
The Future of Voice AI Architecture
Rather than a complete switch to voice-to-voice models, Jordan anticipates the emergence of hybrid architectures leveraging voice-to-voice for context and signal gathering while utilizing specialized agents for different functions within conversations.
Platform flexibility remains essential, with Jordan highlighting the importance of choosing providers that allow easy switching between models as technology evolves. "That's why our approach has always been very modular," Jordan explains when describing Vapi's philosophy. This modular architecture enables Vapi customers to adapt quickly as new capabilities emerge, without being locked into specific technologies that might soon be outdated.
For verticalized voice AI startups, Jordan emphasizes focusing on their competitive edge rather than infrastructure: "I think they should become like the operating system for X industry... voices that are wedged to get in to begin with." This highlights the strategic advantage of using platforms like Vapi to handle the voice infrastructure while vertical solutions concentrate on their industry-specific value proposition.
Optimizing Voice AI Performance
Voice AI optimization ultimately revolves around what Jordan describes as "three variables: performance, latency, and cost." Vapi helps customers navigate these tradeoffs by offering flexibility in model selection and configuration options. Currently, leading solutions on the Vapi platform include Claude 3 Haiku, GPT-4o mini, and ElevenLabs' voice models, with teams selecting configurations based on their specific requirements and priorities.
Vapi's impressive latency improvements come from what Jordan describes as "many, many small innovations over the course of a year and a half," including strategic model selection, end-to-end byte-level streaming, and sophisticated turn-taking models. Jordan explains that Vapi has "heavily instrumented throughout the entire pipeline" to identify and address latency bottlenecks—even accounting for the 400-600 milliseconds of latency introduced by phone lines themselves. These optimizations have made Vapi particularly well-suited for use cases where conversational naturalness is paramount, as even small latency reductions significantly impact conversation quality and user experience.
Evaluating Voice AI Agents with Coval
As voice AI applications become more widespread and sophisticated, the need for robust evaluation frameworks has grown in parallel. During the conversation, Brooke highlighted how Coval emerged in response to this specific challenge: "We started with more generalized evals for any multi-step processes with LLMs and realized that voice was this really hard to test area that was taking off."
Voice AI presents unique evaluation challenges compared to text-based AI systems. With voice, teams need to assess not just the semantic correctness of responses, but also elements like latency, natural turn-taking, handling of interruptions, appropriate tone, and pronunciation accuracy. These factors can make or break the user experience but are difficult to measure systematically.
Coval's evaluation platform helps voice AI developers benchmark their agents across these dimensions, providing actionable insights that go beyond simple accuracy metrics. This is particularly valuable as companies integrate voice agents with platforms like Vapi, helping teams understand how their specific implementation performs in real-world scenarios.
For companies building on voice platforms like Vapi, regular evaluation through tools like Coval provides critical feedback loops that enable continuous improvement. As Jordan observed during the conversation, the voice AI landscape changes rapidly, with performance improvements occurring "every three months" across the stack. Systematic evaluation ensures that teams can take advantage of these advances while maintaining quality and consistency in their voice applications.
Conclusion
As voice AI continues to mature, platforms like Vapi will play an increasingly critical role in bridging raw models and production applications. Whether you're a startup building a vertical solution or an enterprise integrating voice capabilities, understanding the platform landscape—and where solutions like Vapi fit within it—is essential for success in this rapidly evolving space.
The companies that will thrive in voice AI won't necessarily be those building everything from scratch, but rather those focusing on their unique value propositions and core competencies while leveraging platforms like Vapi to handle the complex infrastructure requirements of production voice systems. As Jordan puts it, "I don't think anyone that's not running real-time audio systems should be running real-time audio systems"—a perspective that has guided Vapi's development as a platform that handles these complex challenges so its customers don't have to.
For startups, the question becomes: what is your competitive edge and core competency? If it's not voice technology itself but rather the vertical application of voice, investing limited resources in building infrastructure may be counterproductive. For enterprises making big bets on voice, reliability and scalability become paramount considerations that specialized platforms are best positioned to address.
For companies looking to implement voice AI in 2025 and beyond, Vapi represents an instructive example of how voice platforms are evolving to meet the needs of both startups and enterprises in this dynamic technological landscape.